The importance of data quality in modern analytical environments
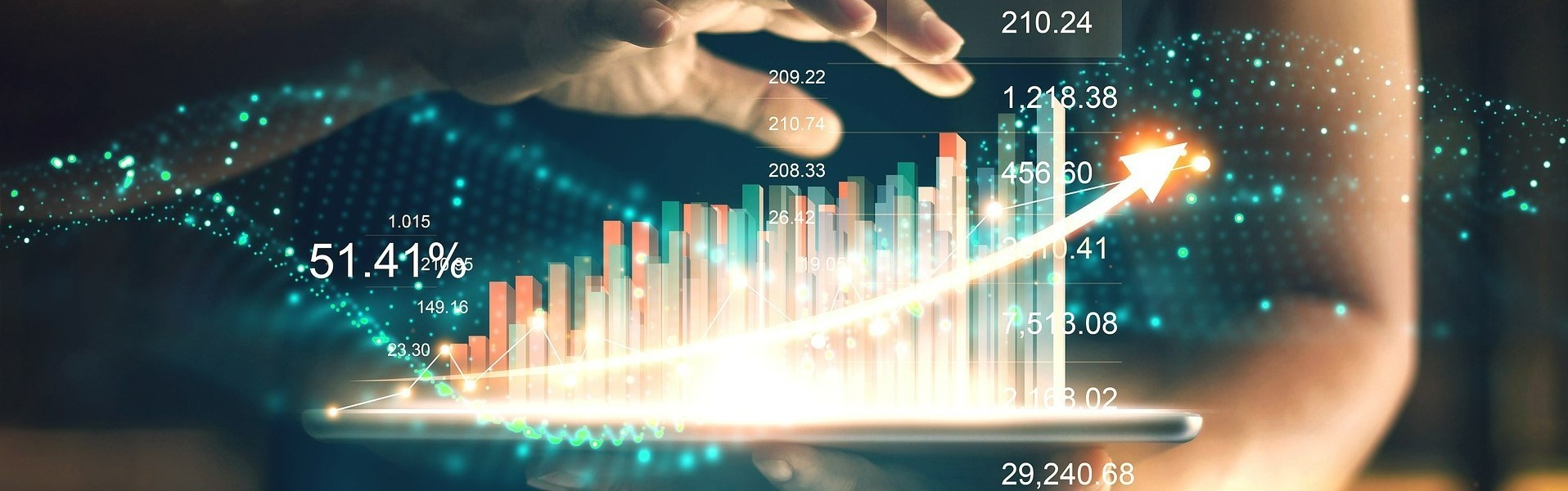
In the world of modern analytics, data quality has become a key success factor. According to the “Data Warehouse and Data Vault Adoption Trends” report by Kevin Petrie and Herbert Stauffer (2023), companies place the highest priority on data quality, ease of use, analytical performance and data governance [1].
The importance of data quality is not exaggerated
Data quality is a multidimensional concept that encompasses, among other things, data accuracy, completeness, consistency, reliability and relevance. Poor data quality can lead to errors in analysis, inaccurate decision-making and a loss of confidence in data systems [2].
Despite its importance, many companies continue to struggle with data quality issues. Inaccurate, duplicate and delayed data continue to disrupt most analytical environments [1]. To overcome these challenges, companies need to reduce their data silos and implement commercial tools to monitor data quality.
Automation, a good solution
Automation plays a crucial role in improving data quality. According to the same report by Petrie and Stauffer, leading companies use commercial automation tools to help them standardize, streamline and repeat data management tasks [1]. These tools can help improve data quality by automating data cleansing processes, identifying and correcting errors, and ensuring data consistency.
Data governance is key
Data governance is another crucial aspect of data quality. It involves defining policies, procedures and standards for data management within an organization. Good data governance can help ensure data accuracy, consistency and security, while promoting confidence in the data [3].
Make data quality your priority
In conclusion, data quality is an essential element of modern analytical environments. To improve data quality, companies need to focus on automation, reducing data silos and implementing effective data governance. By making data quality a priority, companies can get the most out of their analytics investments and make more informed decisions.
References :
[1]: Petrie, K., & Stauffer, H. (2023). Data Warehouse and Data Vault Adoption Trends.
[2]: Redman, T. C. (1998). The impact of poor data quality on the typical enterprise. Communications of the ACM, 41(2), 79-82.
[3]: Khatri, V., & Brown, C. V. (2010). Designing Data Governance. Communications of the ACM, 53(1), 148-152.
Image par Tung Nguyen de https://pixabay.com//?utm_source=link-attribution&utm_medium=referral&utm_campaign=image&utm_content=6701404